Why Deep Learning is important for Enerbrain
By Marco Martellacci
Today it is becoming increasingly difficult to escape the impact of technology on everyday life. The enormous progress that artificial intelligence has brought forward, from deep learning to reinforcement learning, is certainly one of the key factors in this impact.
At Enerbrain we believe that investing in deep learning is very important to improve the energy efficiency of buildings and, consequently, the comfort of our clients. For this reason, we have created a deep learning laboratory within our R&D group.
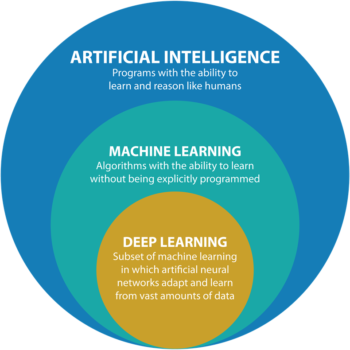
But what exactly is Deep Learning?
This is a subset of a subset of artificial intelligence, which includes most of the rules-based logic systems designed to solve problems. Within the IA, you have machine learning, which uses a set of algorithms to go through the data to make and improve decision making.
In addition, from automatic learning, we get to deep learning, which can make sense of the data by using multiple levels of abstraction. During the training process, a deep neural network learns to discover useful patterns in the digital representation of data, such as sounds and images.
This is why we are seeing further advances in image recognition, machine translation and natural language processing from deep learning. An example is how Facebook can automatically organize photos, identify faces and suggest which friends to tag. Or, how Google can programmatically translate 103 languages with extreme precision.
The most interesting story is for a small company in London called DeepMind, which four years ago uploaded a pioneering algorithm on the ArXiv scientific portal, described in the work “Playing Atari with Deep Reinforcement Learning”.
In this article, Deep Mind showed how a computer has learned how to play Atari video games, which were used 30 years ago, by looking at the screen pixels and processing data related to the score obtained. The result was remarkable because the games and objectives in each event were very different and designed with considerable difficulty even for humans.
The same architecture of the model, without any change, was used to learn seven different games, and in three of them, the algorithm worked even better than a human!
This result has been considered as the first step towards general artificial intelligence—an AI that can survive in a variety of environments, instead of being confined to strict scopes like the game of chess.
It is no wonder DeepMind was immediately purchased by Google, which has long been at the forefront of deep learning research. In February 2015, the aforementioned article appeared on the cover of Nature, one of the most prestigious science magazines. In this article, they applied the same model to 49 different games and achieved superhuman performances in half of them.
The greatest success achieved by DeepMind was to beat the world champion of Go, the most complicated game to manage with a self-learning algorithm.
Enerbrain strongly embraces this technology and is investing in research to apply it to the world of HVAC, in order to achieve results of energy efficiency and comfort of increasingly satisfactory buildings.
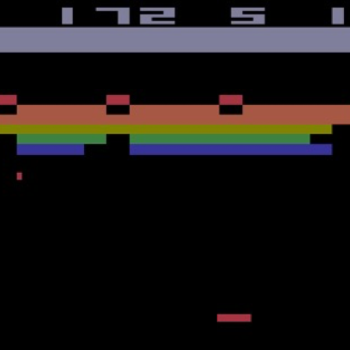